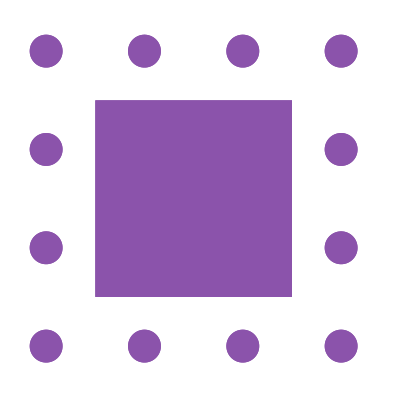
Industry Track Review Criteria
I. Clarity of problem statement (Examples:)
-
Is the problem to be solved well defined?
-
Are the data sources/format/structure clear to the reader?
-
Is the system overall expected input/output clear?
II. Methodology (Examples:)
-
Is the data science/data engineering methodology sound from a foundational point of view?
-
Is it clear what are the parts that come from state-of-the-art/state-of-the-practice vs. any IPR that is contributed by organization (aka technical delta);
-
How you allocated resources to execute this project?
-
How did you managed risk (i.e. of running this project forever vs. having the minimum accuracy/reliability for a MVP release)?
-
How did you timeboxed the different tasks/stages of the project?
-
How did you prioritized the validation of different hypothesis?
-
Why did you choose this method instead of others?
Note: Novelty is not a pre-requisite nor an evaluation criteria in this track
III. Business Impact (Examples:)
-
How this project affected your company?
-
Did this project affected positively your company’s bottomline? How much?
-
How did you measured this outcome? (aka causality)
-
Did this project affect the way you organize your work or your teams?
-
If you asked your company’s CEO to describe this project in 5 words, which words he/she would use?
IV. Lesson's learned (Examples:)
-
If you needed to redo this project again, what you would change AND what you would do again?