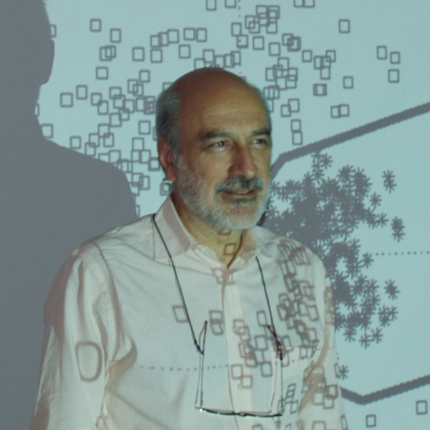
José Carlos Príncipe
Homepage
Bio
Jose C. Principe (M’83-SM’90-F’00) is a Distinguished Professor of Electrical and Computer Engineering and Biomedical Engineering at the University of Florida where he teaches statistical signal processing, machine learning and artificial neural networks (ANNs) modeling. He is Eckis Endowed Professor and the Founder and Director of the University of Florida Computational NeuroEngineering Laboratory (CNEL) www.cnel.ufl.edu. His primary area of interest is time series analysis with adaptive neural models. The CNEL Lab has been studying signal and pattern recognition principles based on information theoretic criteria (entropy and mutual information).
Dr. Principe is an IEEE, IABME, AIMBE Fellow. He was awarded the IEEE Neural Network Pioneer Award, the IEEE Shannon Nyquist Technical Achievement Award from the Signal Processing Society, the EMBS Career Achievement Award, and the Teacher Scholar of the Year from the U. of Florida. He was the past Chair of the Technical Committee on Neural Networks of the IEEE Signal Processing Society, Past-President of the International Neural Network Society, and Past-Editor in Chief of the IEEE Transactions on Biomedical Engineering. Dr. Principe has more than 800 publications and an H index of 88 (Google Scholar). He directed 102 Ph.D. dissertations and 65 Master theses. He wrote in 2000 an interactive electronic book entitled “Neural and Adaptive Systems” published by John Wiley and Sons and more recently co-authored several books on “Brain Machine Interface Engineering” Morgan and Claypool, “Information Theoretic Learning”, Springer, and “Kernel Adaptive Filtering”, Wiley.
Talks
-
A Quantum Theory Inspired Framework for Uncertainty Quantification
Keynote
10-07-2021 - 14:00-15:00
This talk presents our current goal of developing operators inspired by quantum theory to quantify uncertainty in time series and train adaptive models for machine learning. The basic observation is that data projected to a Reproducing Kernel Hilbert Space (RKHS) are functions that obey the properties of a potential field. Therefore, one can directly apply the Schrodinger equation to the projected data and interpret its Hermite expansion in terms of modes over the space of samples that express multi scale uncertainty. This methodology can be used to quantify signal properties and can also lead to methodologies to train signal processing models. We will exemplify the theory with some preliminary results.