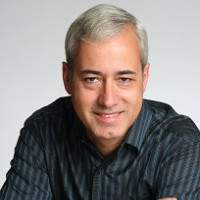
Pedro Domingos
Homepage
Bio
Pedro Domingos is a professor of computer science and engineering at the University of Washington and the author of The Master Algorithm. He is a winner of the SIGKDD Innovation Award and the IJCAI John McCarthy Award, two of the highest honors in data science and AI. He is a AAAI Fellow, and received an NSF CAREER Award, a Sloan Fellowship, a Fulbright Scholarship, an IBM Faculty Award, several best paper awards, and other distinctions. He received an undergraduate degree (1988) and M.S. in Electrical Engineering and Computer Science (1992) from IST, in Lisbon. He received an M.S. (1994) and Ph.D. (1997) in Information and Computer Science from the University of California at Irvine. He spent two years as an assistant professor at IST, before joining the faculty of the University of Washington in 1999. He is the author or co-author of over 200 technical publications in machine learning, data mining, and other areas. He is a member of the editorial board of the Machine Learning journal, co-founder of the International Machine Learning Society, and past associate editor of JAIR. He was program co-chair of KDD-2003 and SRL-2009, and he served on the program committees of AAAI, ICML, IJCAI, KDD, NIPS, SIGMOD, UAI, WWW, and others.
Talks
-
Deep Networks Are Kernel Machines
Keynote
10-06-2021 - 08:30-09:30
Deep learning's successes are often attributed to its ability to automatically discover new representations of the data, rather than relying on handcrafted features like other learning methods. In this talk, however, I will show that deep networks learned by the standard gradient descent algorithm are in fact mathematically approximately equivalent to kernel machines, a learning method that simply memorizes the data and uses it directly for prediction via a similarity function (the kernel). This greatly enhances the interpretability of deep network weights, by elucidating that they are effectively a superposition of the training examples. The network architecture incorporates knowledge of the target function into the kernel. The talk will include a discussion of both the main ideas behind this result and some of its more startling consequences for deep learning, kernel mach
ines, and machine learning at large.